Yi-Zeng Fang, Lung-Hao Lee, and Juinn-Dar Huang.
In Proceedings of the Working Notes of CLEF 2024 – Conference and Labs of the Evaluation Forum.
Abstract
This paper presents a robust methodology for identifying sexism in social media texts as part of the EXIST 2024 challenge. First, we incorporate extensive data preprocessing techniques, including removing redundant elements, standardizing text formats, increasing data diversity by the back-translation, and augmenting texts using the AEDA approach. We then integrate annotator demographics such as gender, age, and ethnicity into our selected transformer-based language models. The rounding technique is used to handle non-continuous annotation values to maintain precise probability distributions. We empirically optimize shared layers across tasks based on the hard parameter-sharing techniques to improve generalization and computational efficiency. Rigorous evaluations were conducted using five-fold cross-validation to ensure the reliability of the findings. Finally, our system was respectively ranked first out of 40, 35, and 33 submissions for Tasks 1, 2 and 3 in the Soft-Soft category setting. In addition, in the Hard-Hard category setting, our system was ranked the first out of 70 submissions for Task 1; second out of 46 submissions for Task 2; and third out of 34 submissions for Task 3. This paper reports our findings in classifying sexism within social media textual content, offering substantial insights for the EXIST 2024 challenge.
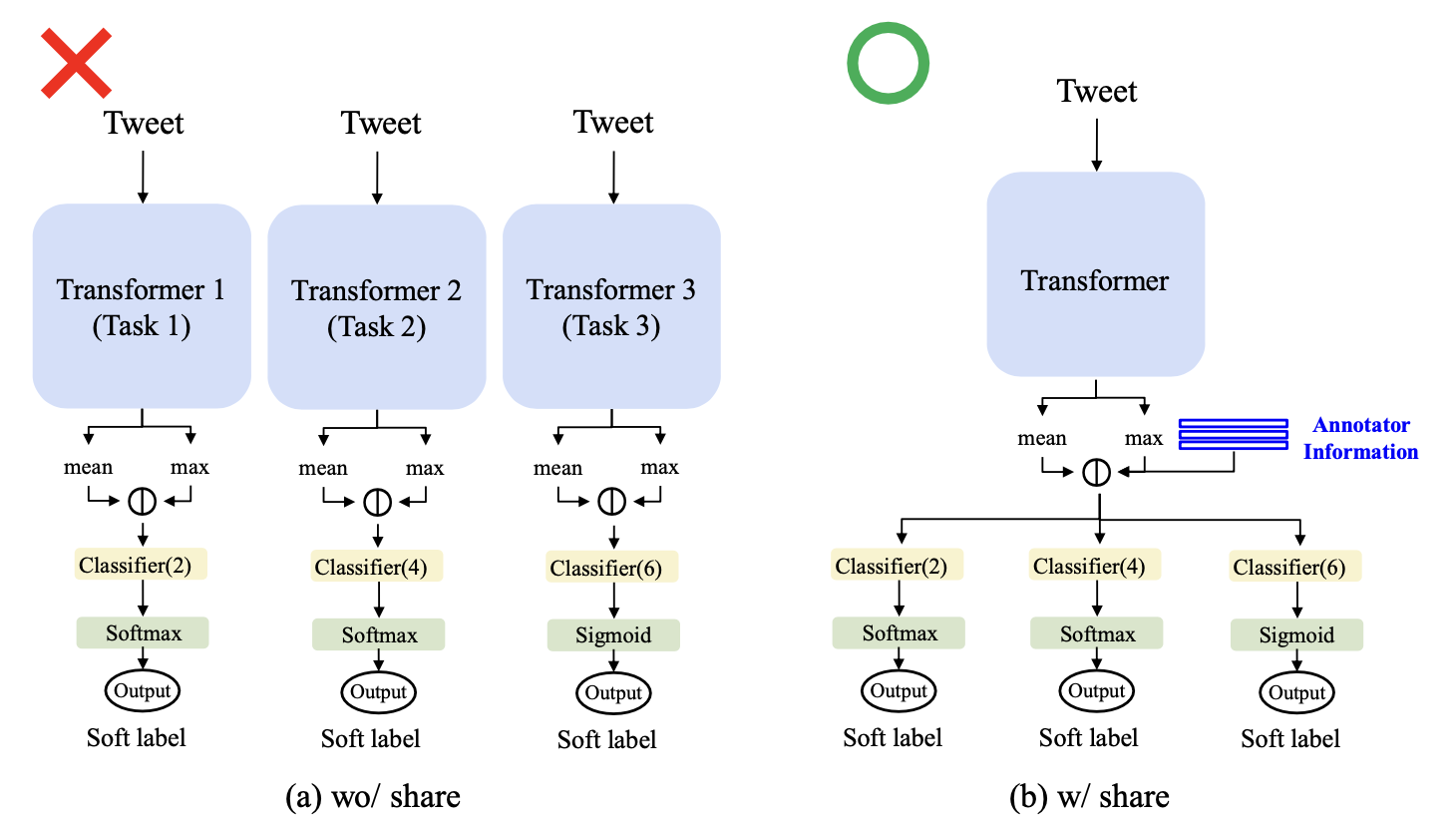