Chao-Yi Chen, Kao-Yuan Tien, Yuan-Hao Cheng, and Lung-Hao Lee. In Proceedings of the 17th International Workshop on Semantic Evaluation (SemEval’23), Toronto, Canada, 13-14 July, 2023, pp. 776-781.
Abstract
This study describes the model design of the NCUEE-NLP system for the SemEval-2023 NLI4CT task that focuses on multi-evidence natural language inference for clinical trial data. We use the LinkBERT transformer in the biomedical domain (denoted as BioLinkBERT) as our main system architecture. First, a set of sentences in clinical trial reports is extracted as evidence for premise-statement inference. This identified evidence is then used to determine the inference relation (i.e., entailment or contradiction). Finally, a soft voting ensemble mechanism is applied to enhance the system performance. For Subtask 1 on textual entailment, our best submission had an F1-score of 0.7091, ranking sixth among all 30 participating teams. For Subtask 2 on evidence retrieval, our best result obtained an F1-score of 0.7940, ranking ninth of 19 submissions.
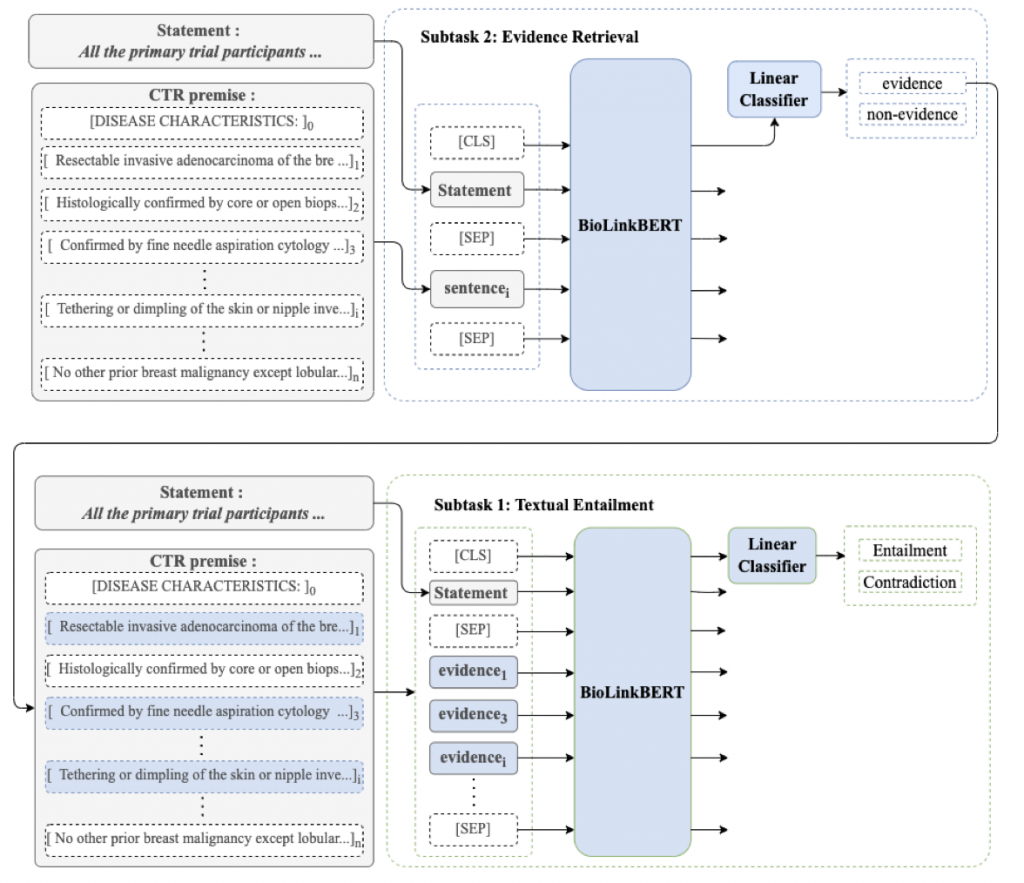