Lung-Hao Lee, Yuan-Hao Cheng, Jen-Hao Yang, and Kao-Yuan Tien. In Proceedings of the 17th International Workshop on Semantic Evaluation (SemEval’23), Toronto, Canada, 13-14 July, 2023, pp. 312-317.
Abstract
This study describes the model design of the NCUEE-NLP system for the SemEval-2023 Task 8. We use the pre-trained transformer models and fine-tune the task datasets to identify medical causal claims and extract population, intervention, and outcome elements in a Reddit post when a claim is given. Our best system submission for the causal claim identification subtask achieved a F1-score of 70.15%. Our best submission for the PIO frame extraction subtask achieved F1-scores of 37.78% for Population class, 43.58% for Intervention class, and 30.67% for Outcome class, resulting in a macro-averaging F1-score of 37.34%. Our system evaluation results ranked second position among all participating teams.
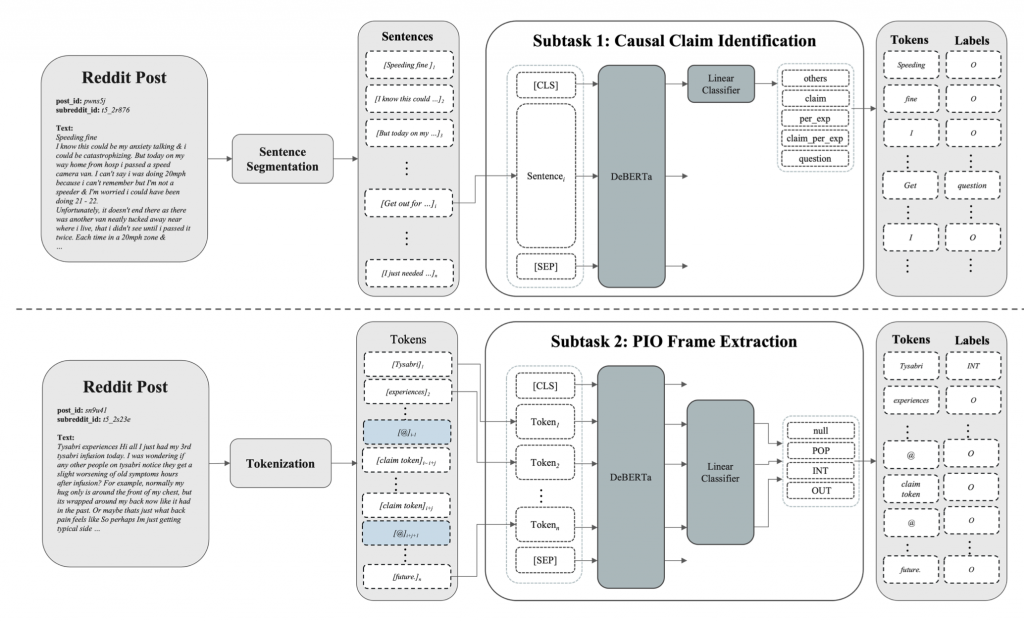